Politeness is briefly defined as “the practical application of good manners or etiquette so as not to offend others.” It is an unspoken set of restrictions on how each person can speak and what they’re allowed to speak about. The purpose of politeness is to avoid behaviors and topics that could provoke hostility in others. Such rules are important in a group of people that want to get along…and then the pigeon shows up.
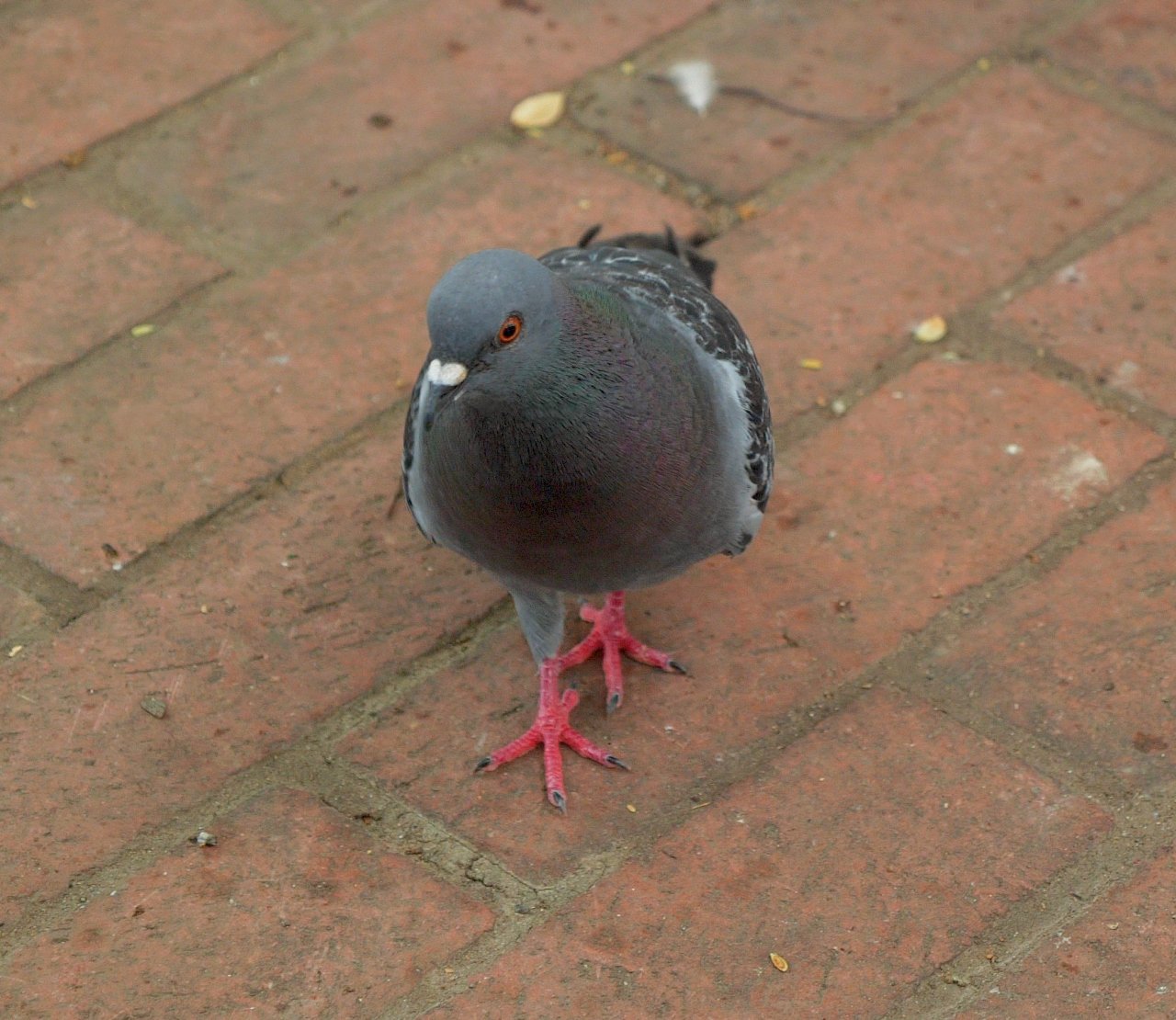
Some say life is like a game of chess. If you’re having a party where people are enjoying games of chess, your door is open, a pigeon flies into your house, lands beside a chess board, and moves a piece…well, isn’t that neat! This pigeon that flew in here is playing chess! You might be kind to the pigeon, giving it a little cup of water and trying to play a mock chess game in return. You and everyone else at the party is being entertained by the chess-playing pigeon even if it doesn’t play chess very well. There’s no harm in letting the pigeon play chess. It’s just doing what everyone else is doing, albeit in a strange way that doesn’t quite fit in with the rest of the group. While some say life is like a game of chess, others say something like this:
“Never play chess with a pigeon. The pigeon just knocks all the pieces over. Then poops all over the board. Then struts around like it won.”
Politeness is self-censorship to avoid conflict. Nature assigns conflict between creatures a very high price; even if you “win” in a fistfight, you’re unlikely to take the victory walk without a limp and a black eye for your trouble. Most people are desperate to avoid conflict because it can hurt them for a long time, be it physically, mentally, or socially. Some people you know may never speak to you again. Your new scar may never stop hurting when something grazes it for the rest of your life. You may fall into a nasty depression from the subsequent fallout and mental flashbacks to the trauma, or from guilt over harm that came to someone that had nothing to do with it. Politeness is a virtue because conflict can have such a high price, but politeness is a nasty vice when conflict is necessary and is prevented.
There is a phenomenon known as the “bystander effect” where individuals are less likely to offer help to a victim in the presence of other people. The two major factors behind it are diffusion of responsibility (in a group of 10, you’re only 10% responsible, so why should you step up?) and compulsion to behave in a socially acceptable manner–otherwise known as “politeness.” If the rest of the group doesn’t step in to stop a bad actor then it’s very likely you won’t either. The “stupidity” of people when they’re in groups is well-known both in psychology and by anyone who observes the behavior of people around them. It’s polite to go along with the group, but it’s not necessarily morally or ethically correct. The first person in a group to respond tends to control the group by doing so, and that person will always be the person who ignores group dynamics, be it by choice (often called “leadership”) or by nature (sometimes socially ignorant, sometimes outright malicious).
Rules governing interpersonal conduct only have value when they represent a net positive; that is, they prevent unnecessary conflict without blocking necessary conflict. Consider someone breaking into your home, jacking your car, or raping your daughter. Most people aren’t willing to extend politeness to this person; in fact, most would happily extend a bullet, a golf club, a baseball bat, or a fist to them instead. Self-defense against a grave threat to your life, liberty, or property is an example of necessary conflict. Demanding that people “be polite” and “play nice” with violent criminals forces them to either become victimized as much as possible or to clearly violate the rules for the sake of self-preservation. This example is presented because it’s painfully obvious, but the same logic applies for lesser “crimes” such as that of the pigeon at the chess party.

Witnessing the poop-laden chess board, the scattered chess pieces and feathers, and the pigeon strutting around without care for what anyone in the room thinks, it becomes obvious to all that the pigeon should have been caught and thrown out right away rather than catered to out of politeness and curiosity. The front door was left open and the host politely allowed the pigeon to set up shop at a chess board without resistance; now there’s a mess and everyone at the party is suffering. Someone could step up, take down the pigeon, and remove it from the party, but no one wants to be the person to break the group dynamic and manhandle the bird, plus at least a couple of “sidewalk superintendents” that like to police group compliance are guaranteed to scold them loudly for being “brutal” to the “poor bird” despite what it has just done.
Eventually, one person decides that enjoying the party is more important than being polite to the creature that is trashing it and that the bite of the politeness harpies can be endured. Grabbing a nearby jacket, this person chases the pigeon throughout the house. The pigeon chaotically runs and flies around to escape, knocking over more things and pooping another time or two in the process, sometimes flying towards people who yell and jump out of the way. The hecklers screech about how the hunter’s interference is only causing the party to become even more wrecked. Others in the group also start demanding that the cruel and fruitless attempts to capture the antagonistic pigeon be halted. All of this takes only a minute or two to unfold and escalate.
Where do we go from here? There are three choices: the pigeon can be caught and removed and the party can resume, the pigeon can be left alone and the party can end prematurely on a sour note as a professional is called to remove the pigeon, or the most polite path can be taken: the party can resume despite the pigeon’s presence. In the polite case, people pretend the stress of the pigeon’s presence doesn’t bother them and the party slowly dies with the pigeon rewarded for its troubles by doing as it pleases. In the early ending case, everyone is unhappy and the pigeon controls the entire house for hours, rewarded for its troubles by doing as it pleases.
In the first and most ideal scenario, the pigeon is removed in a few minutes, the hecklers and those who supported them quiet down so people hopefully forget that their demands were demonstrably the wrong ones, the mess is cleaned up in a few minutes, the party resumes, and everyone walks away an interesting story to tell. The person that removed the pigeon wasn’t polite to the pigeon, the hecklers that followed, or the heckler add-ons that followed again, but in the end everyone was better off because the pigeon was kicked out.
The moral of the story is that sacrificing conflict at the altar of politeness without regard for necessity is a race to the bottom. Another is that people who make and enforce the rules should enforce the rules from the start, not later on after things get messy. None of this would have been necessary if someone had stopped the pigeon from coming into the house in the first place.
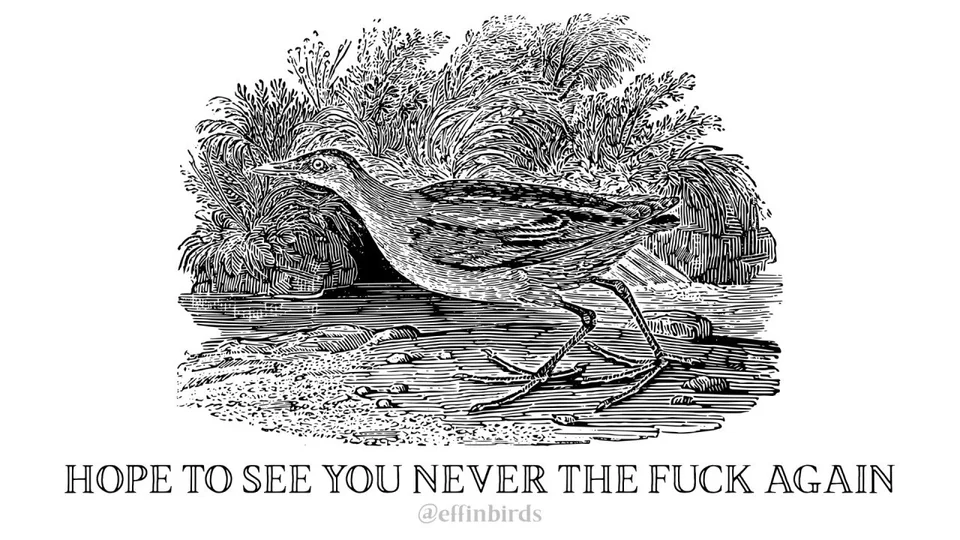